About
In the past years, deep learning methods have taken the medical imaging community by storm. Convolutional neural networks (CNNs) excel at 2D or 3D image analysis, but at the same time, there is a growing realization that not all data is organized on an ordered grid. In many real-world medical applications, for example, genetics and brain imaging, the available data is naturally represented in a non-Euclidean space (e.g., graphs and manifolds). Moreover, data with which the medical imaging community is working contains many translational, rotational, and other symmetries that can be exploited by incorporation in problem design or neural network architectures. For this reason, geometric deep learning has gained significant popularity in the medical domain. This has been reflected in a significant increase in the body of literature using geometric deep learning for challenging medical imaging tasks including image registration, segmentation, and classification.
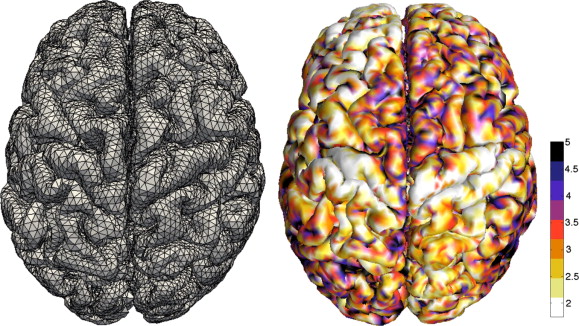
In this workshop, we aim to draw attention to current developments in geometric deep learning for medical image analysis. The main objective of our workshop is to expose the vast richness of geometric structure to be found in medical image data and show how to leverage it in neural network design. We will provide a discussion on the latest state-of-the-art by having invited expert speakers on the topic. We also aim to cover current challenges and opportunities in the area. Our objective is to inspire researchers through a day of exciting keynotes and contributed talks, showing how to design and/or apply methods that leverage geometric structure in imaging problems, e.g., through group convolutions, mesh CNNs, or graph neural networks with geometric priors. The objectives of the Geometric deep learning in medical image analysis (GeoMedIA) workshop are to (a) bring together experts on geometric deep learning in medical image analysis to push the state of the art; (b) hear from invited speakers, and (c) to identify challenges and opportunities for further research.
Contributed papers will be assessed by an expert program committee, and we will provide a best paper award. We will solicit both full papers and short papers. Full papers must contain novel work. They will be published in the workshop proceedings and may be considered for oral presentation. Short papers may contain already published work or work that is under review elsewhere. They will only be considered for poster presentation and will not be included in the workshop proceedings.
GeoMedIA is a MICCAI-endorsed event. GeoMedIA is financial sponsored by the ELLIS Unit Amsterdam, the European Laboratory for Learning and Intelligent Systems. The workshop is further funded by the Dutch Research Council (NWO) through the research programme VENI with project 17290: Context-Aware Artificial Intelligence in Medical Image Analysis.
Program
08.15-09.00 | Registration |
09.00-09.05 | Opening Ceremony |
09.05-10.00 | Keynote Emma Robinson (King’s College London) |
10.00-10.30 | Oral Session 1 |
Scale-Equivariant
UNet for Histopathology Image Segmentation Yilong Yang, Srinandan Dasmahapatra, Sasan Mahmoodi |
|
Group Convolutional
Neural Networks for DWI Segmentation Renfei Liu, Francois Bernard Lauze, Erik J Bekkers, Kenny Erleben, Sune Darkner |
|
10.30-10.45 | ☕ Coffee Break |
10.45-12.00 | Oral Session 2 |
Structured Knowledge
Graphs for Classifying Unseen Patterns in Radiographs Chinmay Prabhakar, Anjany Sekuboyina, Hongwei Li, Johannes C. Paetzold, Suprosanna Shit, Tamaz Amiranashvili, Jens Kleesiek, Bjoern Menze |
|
Detecting Large Vessel
Occlusions using Graph Deep Learning Jad Kassam, Florian Thamm, Leonhard Rist, Oliver Taubmann, Andreas Maier |
|
A Comparative Study
of Graph Neural Networks for Shape Classification in Neuroimaging Nairouz Shehata, Wulfie Bain, Ben Glocker |
|
XEdgeConv: Leveraging
graph convolutions for efficient, permutation- and rotation-invariant dense 3D medical
image segmentation Christian Weihsbach, Lasse Hansen, Mattias P Heinrich |
|
Self-supervised graph
representations of WSIs Oscar Pina, Veronica Vilaplana |
|
12.00-13.00 | Lunch |
13.00-14.00 | Keynote Michael Bronstein (University of Oxford) |
14.15-14.30 | Oral Session 3 |
GeoMorph: Geometric
Deep Learning for Cortical Surface Registration Mohamed A. Suliman, Logan Zane John Williams, Abdulah Fawaz, Emma Claire Robinson |
|
14.30-14.55 | Poster Pitches |
14.55-16.00 | Poster Session & ☕ Coffee Break |
16.00-17.00 | Panel Discussion |
17.00-17.10 | Closing Ceremony & Awards |
17.10- | Drinks Reception |
Accepted full papers
Accepted extended abstracts
Keynote speakers
Prof. Michael Bronstein
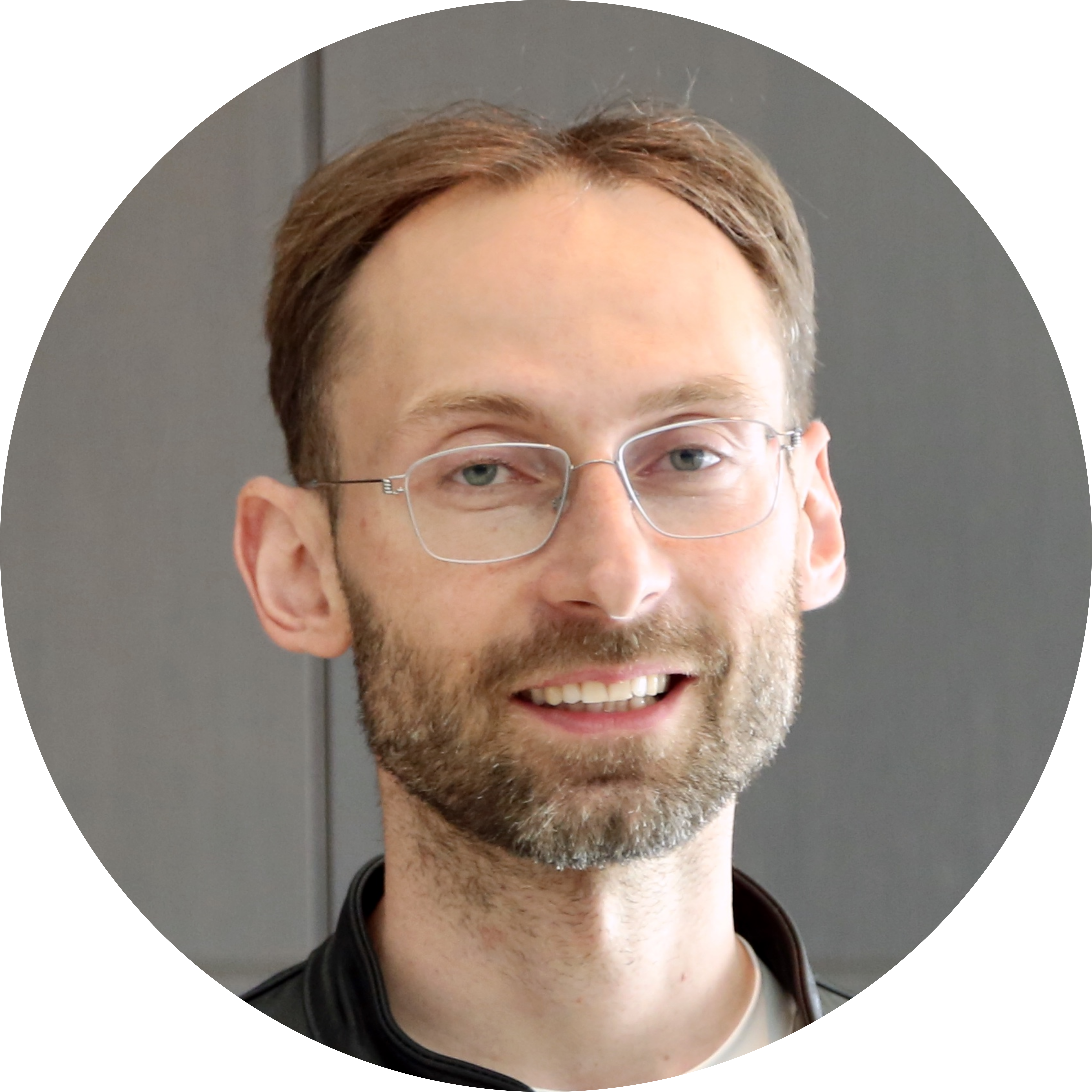
Michael Bronstein is the DeepMind Professor of AI at the University of Oxford and Head. He was previously a professor at Imperial College London and held visiting appointments at Stanford, MIT, and Harvard, and has also been affiliated with three Institutes for Advanced Study (at TUM as a Rudolf Diesel Fellow (2017-2019), at Harvard as a Radcliffe fellow (2017-2018), and at Princeton as a short-time scholar (2020)). Michael received his PhD from the Technion in 2007. He is the recipient of the Royal Society Wolfson Research Merit Award, Royal Academy of Engineering Silver Medal, five ERC grants, two Google Faculty Research Awards, and two Amazon AWS ML Research Awards. He is a Member of the Academia Europaea, Fellow of IEEE, IAPR, BCS, and ELLIS, ACM Distinguished Speaker, and World Economic Forum Young Scientist. In addition to his academic career, Michael is a serial entrepreneur and founder of multiple startup companies, including Novafora, Invision (acquired by Intel in 2012), Videocites, and Fabula AI (acquired by Twitter in 2019).
Dr. Emma Robinson
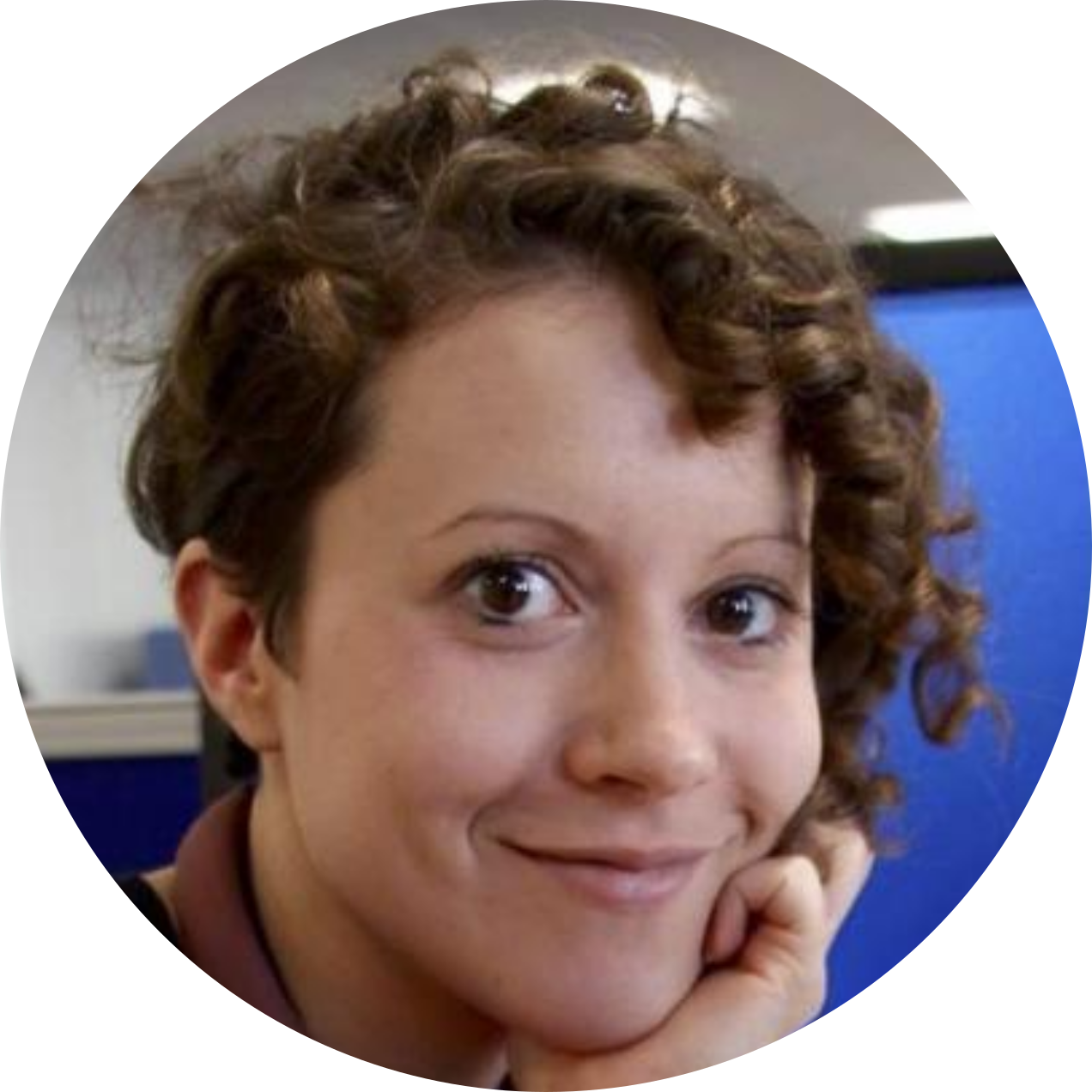
Emma Robinson's research focuses on the development of computational methods for brain imaging analysis, and covers a wide range of image processing and machine learning topics. Most notably, her software for cortical surface registration (Multimodal Surface Matching, MSM) has been central to the development of of the Human Connectome Project’s “Multi-modal parcellation of the Human Cortex “ (Glasser et al, Nature 2016), and has featured as a central tenet in the HCP’s paradigm for neuroimage analysis (Glasser et al, Nature NeuroScience 2016). This work has been widely reported in the media including Wired, Scientific American, and Wall Street Journal). Current research interests are focused on the application of advanced machine learning, and particularly Deep Learning to diverse data sets combining multi-modality imaging data with genetic samples. We are particualrly interested in building sensitive models of cognitive development and developmental outcome for prematurely born babies from data collected for the Developing Human Connectome Project (dHCP).
Registration
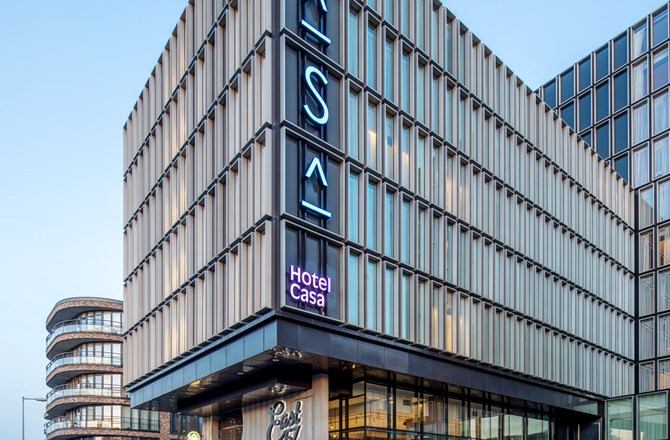
GeoMedIA will be held in the CASA
hotel
in Amsterdam as a one-day workshop. Registration for GeoMedIA is free thanks to our
generous
sponsors. To register for GeoMedIA, please use the form below. Note that we have a limited
number of places
available, and may be unable to accommodate all applicants. Registration will be confirmed
on a first-come
first-serve basis. We also provide a limited number of travel grants for those requiring
support
for their travel to and from Amsterdam. If you want to apply for one of these grants, please
provide the required information in the registration form.
Update November 10: GeoMedIA is at capacity! The registration form has now been closed. Use the form below to be added to our waiting list.
Important Dates
Full paper submission deadline: 4 September 2022
Extended abstract submission deadline: 10 September 24 September 2022
Author notification: 30 September 4 October 2022
GeoMedIA Workshop, Amsterdam: 18 November 2022
Call for Papers
We are happy to invite you to your work to the 1st Geometric Deep Learning in Medical Image Analysis (GeoMedIA) Workshop. Papers can be submitted to one of two tracks:
- Full papers (8 pages + unlimited references and appendices) in the proceedings track and;
- Short papers (3 pages + 1 page for references) in the extended abstract track.
All submissions will be peer-reviewed by an international program committee and accepted papers in the proceedings track will be published in Proceedings of Machine Learning Research (PMLR). The deadline for submissions is 4 September 2022, AOE for full papers and 24 September 2022, AOE for extended abstracts. Papers are to be submitted through OpenReview. All papers will be subject to single-blind reviewing, i.e., no need to anonymze the submission.
We welcome all researchers in medical imaging including mathematicians, computer scientists, bioinformaticians and clinicians. GeoMedIA invites submissions on several themes including but not limited to:
- Applications of geometric deep learning in computer-assisted interventions
- Group convolutional neural networks
- Geometric priors and equivariance in neural networks
- Deep learning on non-Euclidean data structures
- Interpretability of graph neural networks
- Multi-modal image analysis with geometric deep learning
- Domain generalization, transfer learning using geometric deep learning for medical image analysis
- Theoretical analysis of geometric deep learning motivated medical problems
- Fast imaging (e.g., MRI, CT) using geometric deep learning
- Datasets and benchmarks for geometric deep learning in MICCAI
- Efficient annotations using geometric deep learning
- Geometric deep learning for shape processing and analysis
- Geometric deep learning for space-time analysis
For preparation of your submissions, please follow the PMLR guidelines and use the single-column LaTeX template that can be found on this website.
GeoMedIA continues to foster fairness, diversity, and inclusion within its community.
Organizers
GeoMedIA is organized by Jelmer Wolterink, Angelica I Aviles-Rivero, and Erik Bekkers. You can reach us via e-mail or find us on Twitter.
Dr. Jelmer Wolterink (University of Twente) works on novel deep learning methods for medical image analysis. He is the recipient of a prestigious VENI grant from the Dutch Research Council (NWO) for patient-specific aortic aneurysm modeling.
Dr. Angelica I Aviles-Rivero (University of Cambridge) centers on graph-based techniques for (bio-)medical applications, focused on novel functionals (PDEs) with carefully designed priors. Recognitions include an outstanding paper award (ICML 2020) and elected officer (SIAM SIAG/IS 2022).
Dr. Erik Bekkers (University of Amsterdam) develops deep learning methods that respect and leverage the geometric structure of (non-Euclidean) data and problems. Awards include a MICCAI Young Scientist Award (2018) and a VENI grant (NWO) for context-aware AI for medical image analysis.
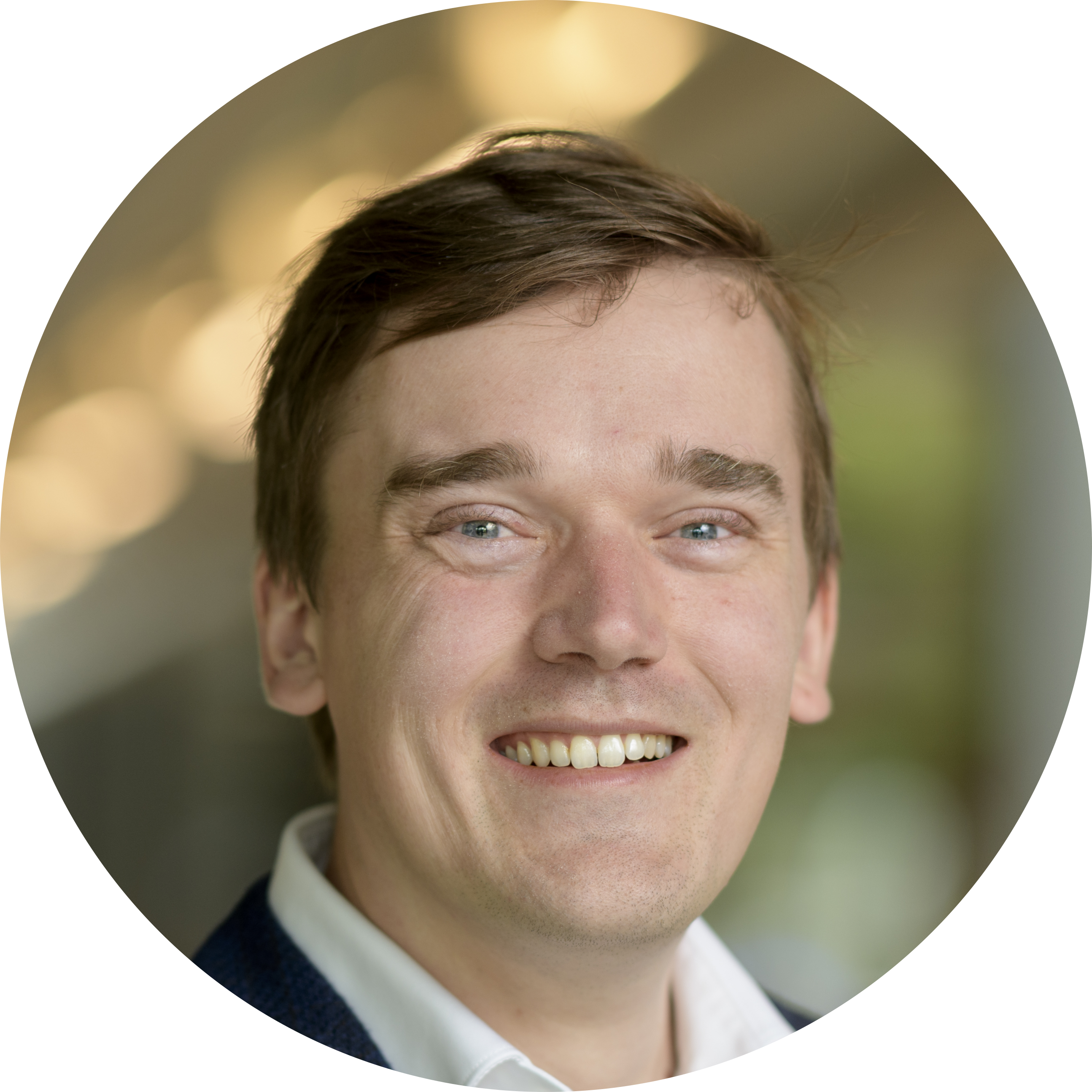
Dr. Jelmer Wolterink
University of Twente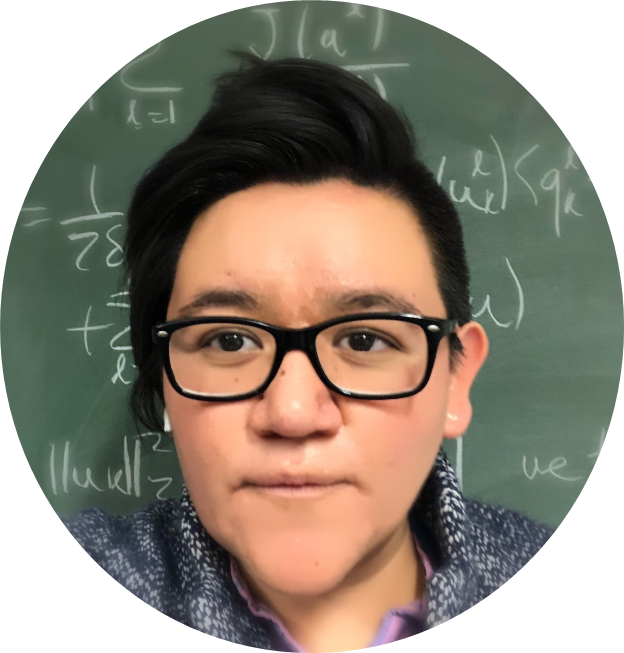
Dr. Angelica Aviles-Rivero
University of Cambridge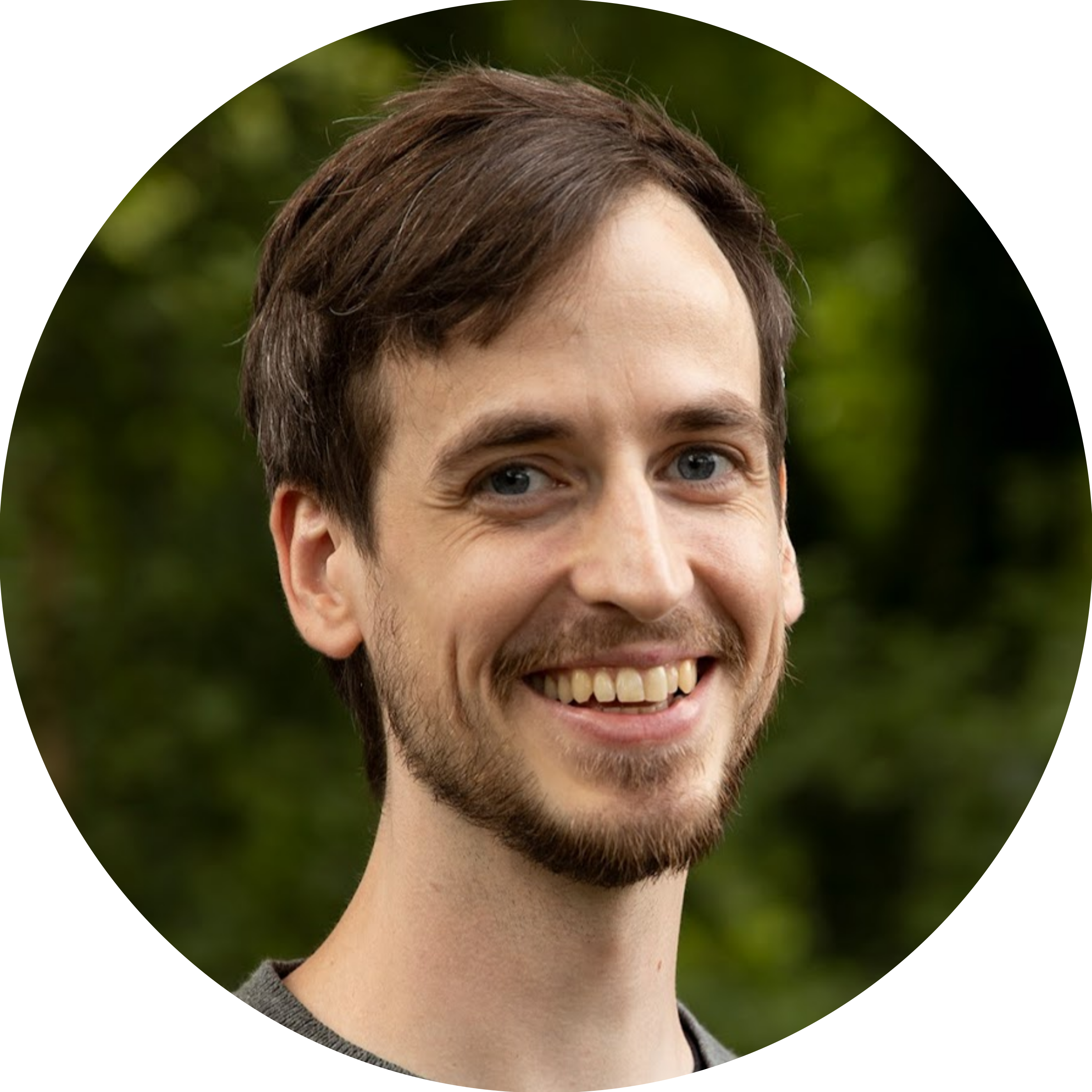
Dr. Erik Bekkers
University of Amsterdam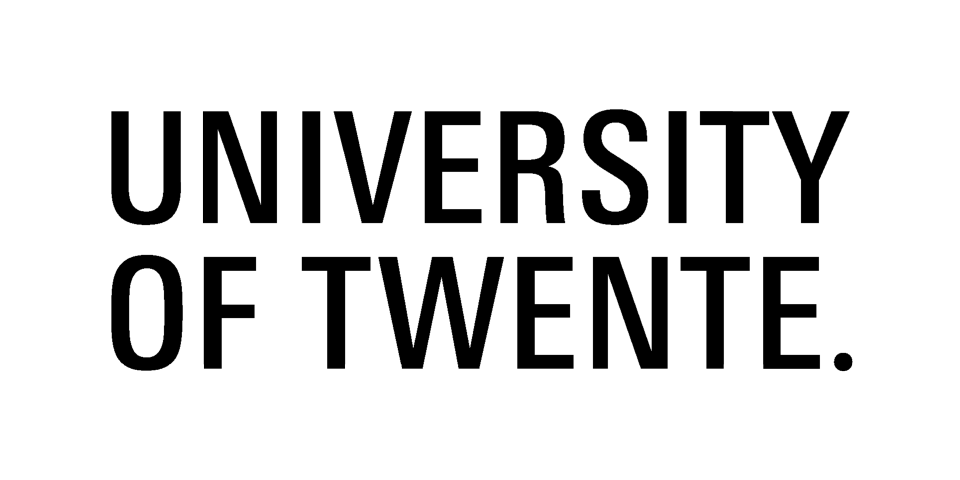
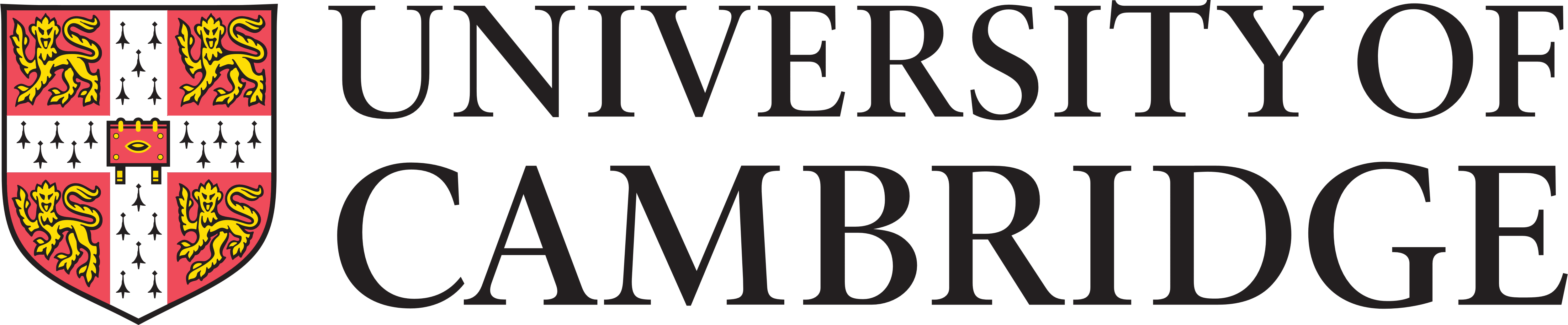
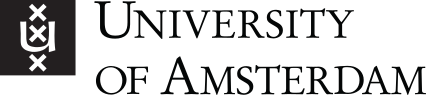